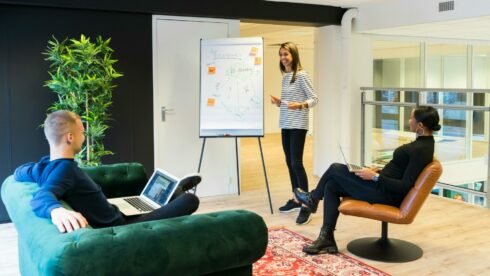
Artificial intelligence (AI) is full of real promise and immeasurable ambition – some realized, most still unfolding.
At the height of our AI ambition, businesses will operate, innovate, and compete by using artificial reasoning, judgment, and creativity to transform, become more efficient and resilient. New capabilities and insights will be revealed that enhance customer experiences, scale competitive advantages, optimize processes, and even predict and prepare for future vulnerabilities.
But to realize our ambitions and see AI reach its full potential, businesses must equally consider the practical aspect required to make it real by operationalizing it.
This means integrating AI into core functions and workflows and ensuring rock-solid governance and human oversight is in place to ensure use cases, insights, ideas, and actions align with the company’s values, goals, and ethics. In addition, AI models should not be theoretical exercises; rather they should be integrated into business processes to deliver tangible value, serving as productivity and effectiveness boosters for every business SME and function.
Let’s explore some of the key challenges and best practices for operationalizing AI, and how it can help enterprises realize their AI ambitions.
Hello AI
Over the last couple of years, as businesses and vendors rapidly shifted to prioritize AI initiatives, they soon encountered the typical complexities associated with launching these projects, such as:
- Data quality and availability
- Integration with existing systems
- Model performance
- A shortage of skilled and expert employees
- Cultural resistance to change
- Ethical and legal considerations.
There is also the question of measurement. What are the right KPIs? What are reasonable business goals? Then of course we must consider end-users. Will they trust the technology, understand how it works, and believe their data is safe? These are all important, early-stage considerations as AI projects are being scoped.
Scoping AI Projects
Operationalizing AI means a real business problem has been 1) identified that AI can materially address or improve and 2) it’s put to work supporting the objective or desired business outcome.
Once a problem has been identified, assessing solutions should include an AI lens that explores improved data models, process enhancement, and potential automation. A consultative approach engaging functional business SMEs and stakeholders should drive the exercise, keeping business objectives at the forefront.
Operations orgs play a unique role, serving as the connective tissue across multiple functions, improving solution design, deployment, and ultimately, scaling the value gleaned from the improvements. New models will require a data assessment and quality assurance processes to boost and maintain effectiveness. Process audits assist with defining gaps and inefficiencies that can be improved with AI. And the all-important change management plan enables business SMEs on the new path forward. These steps aren’t meant to be serial but need to be considered in any solution design.
As the connective tissue, business operations teams are experts at efficiently managing and coordinating the various work streams, vendor relationships, budgets, timelines, problem-solving, change management, etc., required to produce the company’s goods and services. Simply stated, operations is the heartbeat of the business.
A strong business operations team can play a crucial role in the complex process of successful AI deployment and integration through well-regulated, cohesive, and supervised processes. Business operations teams can also oversee detailed project plans and manage stakeholder engagement, establish cross-functional teams full of technical experts, business leaders, and the end-users. When they take the lead on AI projects, outcomes are scaled. Finally, they establish the means to ensure sustained monitoring and feedback to continue to improve the business outcomes over time.
Below are some considerations for operations teams who are supporting their organizations to operationalize AI.
- Define the use case and work backwards. The use-case project owner ideally resides within the operations team in partnership with the business owner seeking to solve the problem. From there you work backward to determine scope, team, timing, etc. A use case for operationalizing AI might be to optimize the routing of delivery vehicles based on traffic, weather, and customer preferences.
- Customize and collaborate. Solving for your specific use case may require a new algorithm, off-the-shelf solution, or sometimes existing capabilities not yet leveraged (another benefit from operations being the connective tissue). It’s not always a new or more complex model that can deliver the most value.
- Look at your toolkit & audit your processes. AI projects often require data cleansing and wrangling tools, data visualization, machine learning frameworks, process changes, model deployment and monitoring tools, some that are not used elsewhere in operations. Defining the new processes and knitting them together isn’t easy. Map out your toolsets and plan for integration, or your use case might get stuck in the training phase longer than you like.
- Analyze for “why.” The line of business owner who owns the use case will need more than just the results provided by a potential ML algorithm or new process. Be prepared to analyze the findings to extract the “why” or create an additional model or process that provides better interpretation.
- Start with low-hanging fruit. Some proven use cases have demonstrated cost savings and added real efficiency to the business. Small process changes and data cleansing or normalization can help teams improve productivity. Automating mundane tasks like issue remediation by providing users with human-readable language. Or using AI-powered virtual agents to manage customer inquiries and basic support requests.
- Latency matters. Evaluate your cloud architecture—can it scale to support your new AI algorithm? Ensuring your cloud architecture can deliver the latency required to meet business needs is critical.
- Responsible AI matters most. Therein lies one of the biggest challenges. AI must be accountable, inclusive, reliable, safe, fair, transparent, private, and secure. Responsible AI implementation has considered the company’s values, policies, and standard operating procedures, as well as legal and moral implications around the lifecycle of AI – fostering a culture of trust and ethical decision making. Successful AI requires we humans.
By empowering business operations teams to drive strategies to overcome some of the mentioned challenges, businesses can improve their chances of successfully introducing AI products and capabilities throughout the entire business.
Operationalizing AI is not a one-time event, but a continuous process of learning, adapting, and improving. It starts with leaders promoting a culture of innovation and willingness to try new things. It requires trust between teams, a collaborative mindset, and a robust infrastructure. By operationalizing AI, global businesses can realize their AI ambitions and benefit from AI’s limitless potential, making them more competitive and driving business value.
You may also like…
How to maximize your ROI for AI in software development
Q&A: The disconnect between the C-Suite and IT practitioners on AI readiness