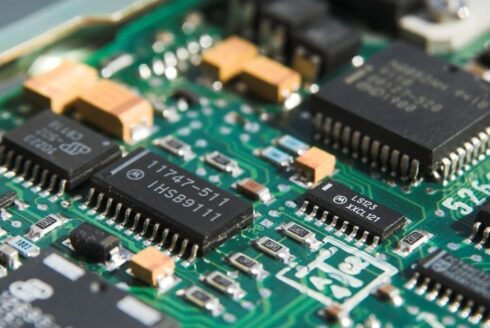
Powering everyday devices — from cars to smartphones — the semiconductor chip demand was high before the pandemic. When it comes to producing these vital pieces of technology, there are two critical components: having a chip design that provides advanced levels of functionality and sustained performance to power a range of devices, and a quick and efficient production process that can accelerate chip makers’ time to market. With the technology industry’s rapid pace of innovation and digitization, demand for even more advanced chips has only accelerated. Paired with today’s ongoing chip shortage, demand is becoming increasingly difficult to satisfy. In fact, according to consulting firm AlixPartners, the global automotive industry alone lost nearly $110 billion in revenue in 2021 due to the on-going chip shortage. This issue has also become a priority item on the White House agenda given the chip shortage’s significant economic impact on the nation’s GDP growth and job stability within the manufacturing industry.
To address this growing need and demand, manufacturers have aimed to speed EDA (electronic design automation) workloads to accelerate the chip design process. With the ability to process simulations, enable physical design and create verification processes throughout the workflow, new EDA tools have helped chip makers produce more advanced chips while accelerating their time to market. But as chip designs have grown in capability and complexity, so do the EDA workloads needed to bring them to life. Today, the increase in EDA workloads is driving huge demands for additional compute and storage resources and running these jobs at maximum efficiency is critical.
But optimizing EDA workloads is not as simple as it seems. Because EDA environments tend to have high levels of concurrency and parallelism, they also require high levels of compute and processing power to enable the 10s-100s of thousands of runs, with many jobs accessing the same project folders and performing huge amounts of concurrent I/O operations. As a result, manufacturers have taken various approaches for enabling EDA, leveraging on-premise infrastructure or the public cloud – but each has their own set of unique benefits and drawbacks.
On-Premise Infrastructure Benefits and Concerns
The benefit of using on-premise infrastructure for EDA workloads lies in its ability to provide chip makers with complete control over their sensitive chip design and manufacturing process data. Chip makers can also leverage their cybersecurity practices and levels of data access to prevent potential data breaches and cyber attacks.
Storage and infrastructure performance remains a key concern. Because EDA workloads are often required to perform metadata, read and write operations in one or many files in parallel from shared storage, it requires a data layer that can keep up with the demanding, concurrent workloads that legacy on-premise storage infrastructure often can’t provide. If the data layer performs slowly, companies risk delayed EDA jobs, longer runtimes and ultimately, higher costs.
Additionally, data storage and the ability to scale storage performance is another critical capability for EDA environments. For example, during the design process of EDA workloads, compute cores scale independently of storage, making it difficult for manufacturers to manage the high number of cores. Because of this, storage performance needs to scale linearly to mitigate the effect of storage-related slowdowns and bottlenecks. Other challenges associated with data storage for EDA workloads include the use of siloed data warehouses, which makes it difficult to coordinate or collaborate remotely on EDA workflows; storage bottlenecks for RTL simulations and storage solutions that lack the agility to manage millions of small files each with their own heavy metadata descriptions.
While it’s possible to upgrade and maintain on-premise infrastructure to enable EDA workloads, doing so is often costly, time consuming, and even impractical to complete based on product maps and timelines.
Leveraging a Public Cloud Approach
As a solution, many organizations have turned to the public cloud for their EDA workloads. With its practically endless availability of CPU and GPU resources, engineers can run many EDA workloads at once without being limited to on-premise data center resources, giving them the ability to achieve faster time to results, and ultimately more business value.
But this can pose new concerns for chip makers around security and data control. More specifically, moving intellectual property and chip design data from the datacenter to the cloud can expose businesses to many new risks such as IP security, data sovereignty and cloud lock-in. Data mobility is another important factor to consider; EDA workloads require high compute resources to run design jobs and manufacturing processes 24/7, but manufacturers require the ability to quickly provision infrastructure on demand to drive faster time to market. While using public cloud providers can provide manufacturers with the computer resources they need for modeling and simulation processes, there can be legal ramifications for moving intellectual property for chip design and manufacturing because of issues around control and data locality. Despite these challenges, using the cloud remains to be a beneficial option for chip providers, offering manufacturers significant advantages in compute and data processing power that can meet the unique needs of EDA workloads.
Storage Considerations for Your Own EDA Workloads
Whether leveraging on-premise infrastructure or cloud platforms for EDA workloads, it’s important to consider how infrastructure performance will impact the chip design process. Investing in the right infrastructure with storage capabilities that can scale out non-disruptively and combine file and object storage protocols with the low latency, massive parallelism, and high throughput capabilities can be beneficial for enabling EDA environments. With the proper infrastructure and storage capabilities in place, manufacturers can efficiently design and produce the chips that are at the heart of all smart devices – faster, at scale and with greater return on investment.