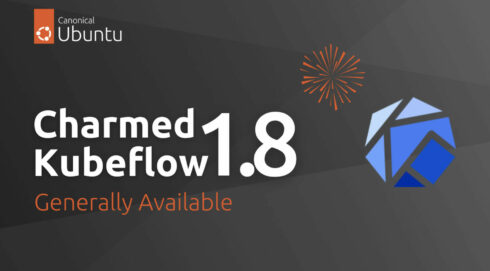
Canonical, the publisher of Ubuntu, has released Charmed Kubeflow 1.8, the latest version of the company’s managed version of the Kubeflow project, which is an open-source MLOps platform.
This platform enables the development and deployment of AI/ML models and is compatible with various cloud environments, including hybrid and multi-cloud setups.
The new release also introduces the capability to run AI/ML workloads in isolated, air-gapped environments, offering an added layer of security. This feature is beneficial for organizations in regulated industries or projects dealing with sensitive data.
The inclusion of offline capabilities streamlines the machine learning workflow by allowing users to execute most tasks within a single tool, eliminating the need for connecting multiple tools and ensuring compatibility, according to the company in a post.
Charmed Kubeflow 1.8 introduces enhancements that enable users to customize their MLOps platform according to their preferences. This includes the ability to add any image within Jupyter Notebooks, providing flexibility for professionals to use their preferred tools and libraries.
This customization feature allows users to focus on developing machine learning models rather than managing tooling. Unlike the upstream project, Charmed Kubeflow’s capability to plug in and out tools or components for specific use cases is unique, according to the company.
“Kubeflow as a project was designed to run AI at scale. It is able to run the entire machine learning lifecycle within one tool. Kubeflow Pipelines are the heart of the project, since they are specialised in automating machine learning workloads. This is one of the reasons organisations that are looking to scale AI projects prefer Kubeflow over its alternatives,” Andreea Munteanu, product manager at Canonical for Machine Learning Operations wrote.
Charmed Kubeflow leverages the advancements in Kubeflow Pipelines 2.0 from the upstream project, streamlining the automation process. While some of its capabilities, like directed acyclic graphs (DAG), have already been available in beta, Kubeflow 1.8 introduces additional features. Kubeflow now abstracts the pipeline representation format, enabling it to run on any MLOps platform. This abstraction facilitates smoother migrations from the upstream project to distributions or tools providing enterprise support, security patching, or timely bug fixes.