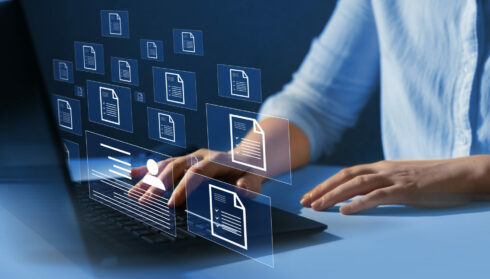
After devoting much of 2023 to AI pilots and experiments following the debut of ChatGPT and GenAI, AI-minded organizations are now working to scale their AI initiatives to capitalize on their early but limited successes. Their goal? To integrate advanced AI capabilities across their operations for greater efficiency, innovation, and competitiveness. An April KPMG survey found that 39% of CEOs are ready to industrialize their AI pilots across multiple business functions or units this year.
Scaling AI means leveraging vast volumes of data to train an organization’s AI models. But following the passage of the EU AI Act in March and, more recently, the multinational government and company commitments made to AI safety at the AI Seoul Summit, global enterprises risk potentially crippling penalties – up to $37.9 million (€35 million), or 7% of their worldwide annual turnover via the EU AI Act alone – if they fail to safeguard their AI operations and the sensitive data that fuels them.
However, mature, AI-forward companies are already differentiating themselves from competitors by managing their AI operations responsibly – regardless of what is or will be expected from a regulatory standpoint. As unbinding as their pledges to responsible AI may be, major tech companies like Microsoft, IBM, Salesforce, and Google have collaborated with governments on regulatory frameworks, investing in tools and processes to enable AI security and transparency and outlining principles for building trust.
All enterprises, big and small, should do the same if for one reason: to cater to the customers who demand it. But where to start?
Putting Responsible AI into Practice
Truly institutionalizing responsible AI requires a comprehensive approach to all AI operations for every model developed and deployed within the organization. This means bringing full data governance into every step of the AI model lifecycle – from initial training and regional fine-tuning to local deployment and ongoing monitoring. (According to recent S&P Global research commissioned by Vultr, data governance was named the biggest obstacle to achieving AI maturity.)
Full data governance comprises the following key components:
-
Federated Data Governance: This requires defining clear roles – e.g., data stewards, governance administrators, etc. – across business units, creating business-friendly data terminology, assigning ownership to data products/domains, and instituting governance councils that can align practices organization-wide.
-
Data Quality and Lineage: High-quality data is essential for reliable AI model performance. This can be achieved by implementing data quality rules across hybrid environments, traceability of data lineage and provenance, and automated AI/ML-powered data quality checks.
-
AI/ML Model Governance: This includes bias testing, model monitoring, and enforcement of fairness, transparency, privacy, and other ethical AI principles. It also involves leveraging AI/ML to automate model validation, drift detection, and compliance checks.
-
Data Security and Privacy: Security is critical when processing sensitive data, particularly at the edge where various jurisdictions uphold differing requirements. Enterprises must implement data access control, encryption, and privacy-enhancing techniques – e.g., differential privacy and federated learning – to ensure compliance with local, federal, and regional regulations such as GDPR and CCPA.
Data Governance in the Hub-and-Spoke Operating Model
Scaling AI operations efficiently and responsibly is possible through a hub-and-spoke operational paradigm that centralizes models’ initial development and training while enabling localized fine-tuning and deployment.
It calls for the following:
-
Establishing an AI Center of Excellence: This will house the organization’s top data scientists and serve as the centralized core for developing and training AI models.
-
Drawing on Open-Source Models: Tapping open-source AI models available in public registries supports transparency, explainability, security, and privacy – not to mention many business benefits, including cost savings.
-
Training on Proprietary Data: Training open-source models on proprietary company data allows enterprises to create models that leverage their unique, highly valuable intellectual property to support their business objectives.
-
Utilizing Private Model Registries: All proprietary models are containerized and stored in a private registry to protect the intellectual property they now contain. This “walled garden” is then made available to localized data science teams, wherever they may be.
-
Fine-Tuning by Regional Data Science Teams: Geographically dispersed data science teams pull proprietary models from the private registry by setting up Kubernetes clusters in edge locations where the containerized AI models are then deployed. Here, the data scientists fine-tune the models on regional or local data to address specific regional characteristics while maintaining compliance with local data governance requirements.
-
Building Vector Databases for Retrieval Augmented Generation (RAG): Data science teams store relevant (and often highly confidential) data they wish to exclude from the core training data as embeddings in vector databases, improving the quality and accuracy of the model’s outputs. Storing such data as embeddings allows data scientists to incorporate current information from external sources that may not be present in the original training data, render the model’s outputs more transparent by providing sources for the retrieved context, and reduce the need to retrain the model as new data is made available.
-
Localizing Deployment, Inference, and Monitoring: Fine-tuned models are moved into production, where local data science teams use observability tools to continuously monitor model performance. Such localized monitoring allows the data scientists to quickly adapt the models to any changes or anomalies in the local environment and correct any instances of drift or bias.
Data Governance at Scale Demands Purpose-Built Platform Engineering
The only way to get to mature AI is through the discipline of the hub-and-spoke operating model, which ensures proper data governance at every step. This is easy to do when looking at any one model in isolation. However, enterprises with mature AI operations will likely have dozens or even hundreds of models in production at any given time. Hence, they need the automation and repeatable processes that platform engineering solutions – purpose-built to support distributed AI – can provide. Platform engineering solutions built for AI operations can and must put proper data governance at the foundation of distributed AI operations.
Data Governance: Good for Business; Good for Humanity
By combining complete data and AI compliance with the hub-and-spoke operating model, organizations can ensure the integrity of their AI operations at scale across all regions around the globe, protecting them from regulatory infractions. But the journey toward responsible AI isn’t just about avoiding steep regulatory fines. Organizations that can bake ethical AI into their businesses’ DNA will foster innovation unfettered by disruptive regulatory oversight. Doing so will create an advantage over competitors trying to toe the regulatory line.
It will also demonstrate the organization’s dedication to creating a future where technology serves customers and humanity in ways that best serve everyone’s interests.