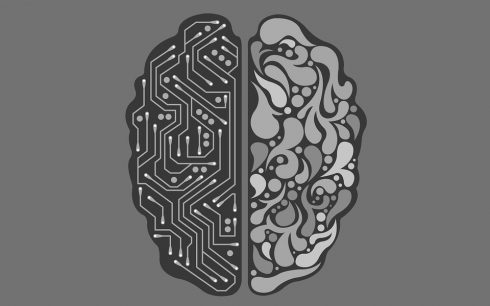
“AIOps” is a term that has been buzzing around for a while, which is short form for “Artificial Intelligence Operations” (much more of a mouthful), and encompasses within it two dominant tech trends:
- The data explosion in recent years which lays the foundation for extracting intelligent, models, patterns and recurring trends; and
- The significant progress achieved in the area of artificial intelligence as a whole.
Both of these together are a very promising and powerful combination for building predictions, and eventually automatic actions to be taken based upon these models and predictions, such as intelligent remediations or other critical fixes.
But the big question remains – do robots really have what it takes to completely take over the role of the human operator?
The AIOps Opportunity
So why is the term thrown around so often? It’s undoubtedly due to today’s large-scale, hyper-growth technology stack reality. The trillion dollar cloud market, which heralds the nearly ubiquitous shift away from centralized IT to more distributed systems and microservices for the inherent innovation and agility benefits it brings with it, also introduces tremendous complexity to formerly simpler systems’ engineering challenges.
The AIOps gospel at its core proclaims that we’re in an era of machines so smart, so capable of self-learning that they can replace humans for troubleshooting even the most complex of engineering problems. The assertion is that there are many similar problems that have occurred before, models that can be created from this data, and workflows and automation that can be applied to fix outages, production failures and virtually any other engineering issues that arise. But is this so?
I’m not so sure. While there is plenty that can be learned from previous mistakes to provide much greater clarity and insights into our systems, in the age-old DevOps adage, correlation does not always == causation. Basically, the relations that machines might believe make a lot of sense – seeing as these are very cut and dry binary deductions; they may not always be truly accurate in the real world, and even more so when it comes to highly complex system operations. This often results in false negatives and false positives from things as simple as insufficient testing of services or misconfigurations, respectively.
“AIOps” just might be a great marketing term coined by vendors who want to quickly tap into the pain points of maintaining modern complex systems, and some of it may actually be quite useful but I believe there is a better, more practically applicable term that’s starting to catch on.
Enter Change Intelligence
What if there was a way to achieve tangible automation to real complex system engineering problems without eliminating the humans in the process?
There certainly is value in leveraging machines for what they’re good at: correlating patterns, understanding recurring trends, providing a real dry and exact analysis of change that occurred in systems, while empowering the humans in the process to understand the correct actions and measures to be taken upon this intelligence.
Change intelligence basically plays to the strengths of both the humans and machines in the process, where each plays a critical role. This is particularly true in high-pressure environments – such as during production outages and failures that require rapid remediation, based upon true (machine) insights. We say do away with the “A” in AIOps – no need for artificial anything, but rather just focus on operations intelligence. This is achieved by understanding what recently changed, and where, and how this is impacting our systems. Then provide the human a play-by-play runbook to run based on common system failures.
There is certainly enough data to analyze to learn from common failures and outages, especially those that are based on known system engineering problems. However “automating out” the humans from the process doesn’t seem completely feasible in engineering just yet, and this isn’t exclusive to AI-powered intelligence and operations.
This is often true when it comes to engineering. Even the most advanced of engineering organizations, who live and breathe DevOps culture, might have a more manual process for continuous deployments (particularly with major changes or versions), to ensure that a human operator oversees an important code change. Even with the advancements of CI/CD, workflow and pipeline automation, there have been many issues that arise with full end-to-end automation processes that are completely handled by machines. Today’s more common practice involves humans pressing the last button for major deployments.
So why ask machines to solve your problems when intelligent humans can probably do this better and more quickly if they have the right tools?
To put it simply: what engineers want is to understand what changed and why in their systems, in real time. They are not asking, nor do they trust, machines to be resolving major incidents for them. When it comes to major incidents, by definition, they are unique black swan events, so the prior data AI leverages to identify patterns is mostly useless. However, in some scenarios identifying anomalies and recent changes is actually quite useful. What changed just moments ago could point to the root cause much more quickly.
Bottom Line: Managing AI vs Managed by AI
So to answer the previously posed question – no, I do not believe AIOps and machines in their current state can deliver on this promise. That’s not to say there haven’t been tremendous strides in automation and even machine learning. Perhaps we’ll arrive at a future where the job of operators becomes managing and maintaining AI, instead of the systems directly. But we are far, far away from AI making better value judgments than engineers in the world of operations. Change Intelligence is real and it’s here today, and there are plenty of amazing software vendors helping engineers better understand the changes happening within their complex systems.