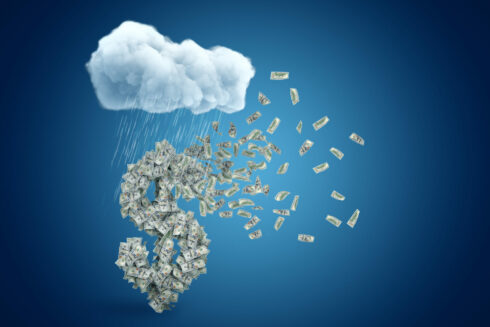
Overprovisioning remains an issue for infrastructure teams manually managing Kubernetes clusters in public clouds. While not unexpected, it is still frustrating to see because it is completely avoidable using automation, according to the third annual Kubernetes Cost Benchmark Report published today by Kubernetes automation provider CAST AI.
The report found that the average CPU utilization across Kubernetes clusters is at 10%, down from 13% last year. Memory utilization was reported at 23% — a modest 3% increase from the previous year.
In the research, a way to save on costs in by using Spot Instances, which are discounted resources that runs on unused compute power. As an example, the report found that clusters partially leveraging Spot Instances can reduce compute cost by 59%; clusters using only Spot Instances saw a savings of 77%.
“When we onboard new customers, they have two ‘ah-ha’ moments,” said Laurent Gil, president and co-founder of Cast AI. The first is when they enable automation and see immediate cost savings via workload rightsizing, bin-packing, and instance-type selection. The second is when they realize automation isn’t just saving them money, it’s freeing them up to up-level their creative thinking and spend more time solving mission-critical business problems.”
This year’s report also examined GPU availability and pricing. According to the report, GPU availability varies by cloud provider. Cast AI analyzed different regions and Availability Zones to find where specific GPU chips are most available, and “compared the cost of running workloads on some of the hardest-to-get GPUs.” It found that companies that can move their workloads to more cost-effective sites around the world had 2x to 7x savings compared with average Spot Instance pricing, and from 3x to 10x savines compared with On-Demand Instance pricing.
According to Cast AI, the report is based on an analysis of 2,100+ organizations across Amazon Web Services (AWS), Google Cloud Platform (GCP), and Microsoft Azure (Azure) between January 1 and December 31, 2024. This analysis excludes clusters with fewer than 50 CPUs and focuses on data collected before these organizations used Cast AI’s automation.