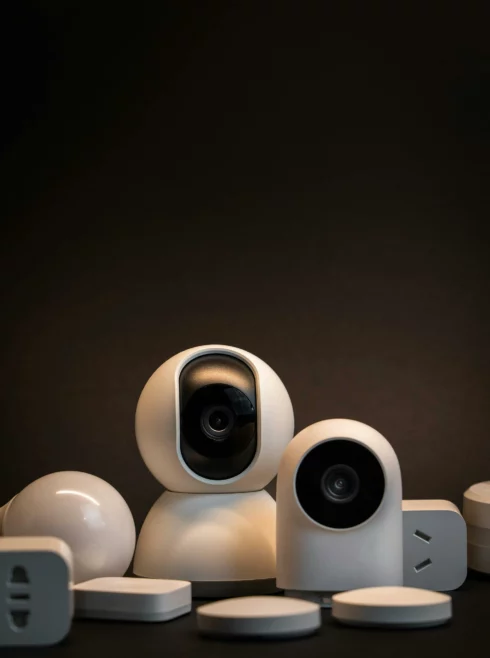
It seems like just yesterday when the Internet of Things (IoT) accelerating digital transformation became the topic of conversation in developer and industrial communities. Now, with AI advancing at breakneck speed, the conversation has shifted to AI-enabled IoT—the Artificial Intelligence of Things (AIoT). This technological convergence between AI and IoT is the powerful new paradigm driving real-time AI-driven automation. It’s fundamentally reshaping how businesses approach IoT data strategies, creating opportunities for those agile enough to adapt.
Traditionally, IoT data processing followed a collect-store-analyze model. Data would flow from sensors to centralized repositories where it would eventually be processed—often hours or days after collection. This approach, while functional, limited the strategic value of IoT implementations.
The integration of real-time AI capabilities marks a profound shift from reactive to proactive intelligence. Today’s leading organizations are exploring the deployment of AI algorithms at the edge to enable instant analysis and automated decision-making. As edge use cases continue to expand, Gartner projects that enterprises will move toward the adoption of edge AI. This evolution transforms IoT networks from simple data collection mechanisms into autonomous systems capable of independent action.
Consider manufacturing environments where equipment failures can cost millions in downtime. Traditional approaches might detect anomalies in operational data, generate alerts, and wait for human intervention. In contrast, AI-driven systems can identify pre-failure patterns, automatically adjust operational parameters, and schedule maintenance before critical failures occur—all without human involvement.
The Architecture of Immediacy
IoT has come a long way since Mike Freedman and I co-founded Timescale in 2015. We’ve watched the convergence of AI and IoT transform from an interesting possibility to a business-critical imperative for forward-looking enterprises in the industrial, energy, and logistics space.
We realized that real-time data intelligence demands a fundamental rethinking of IoT data architectures. Four key components are emerging as essential to those architectures:
Edge Intelligence. Processing power is increasingly moving to the edge of networks, where data originates. This distributed computing approach reduces latency and enables instant decision-making where it matters most.
AI-optimized data storage and transmission. AI can determine which data needs to be stored, transmitted, or discarded, reducing data overload and storage costs. Adaptive data compression and prioritization techniques ensure optimal cloud and edge resource utilization.
Adaptive Learning Systems. Today’s most effective IoT implementations incorporate AI systems that continuously learn from new data, refining their models and improving accuracy over time.
Closed-Loop Automation. The true power of real-time AI lies in its ability to close the loop between insight and action, creating systems that not only detect issues but resolve them autonomously.
When Machine Learning Becomes True Intelligence
Unlike traditional machine learning (ML) models that require periodic retraining, AI models can learn from new IoT data and adapt dynamically. The evolution from traditional ML approaches to modern AI solutions for IoT data creates new insight pathways, such as those profiled below.
Multimodal Data Integration. Modern AI can synthesize diverse data types (vibration, acoustic, thermal, visual) simultaneously to develop a comprehensive understanding of equipment health. This provides richer contextual awareness than single-sensor machine learning models.
Unsupervised Anomaly Detection. Advanced AI models can identify novel failure patterns without being explicitly trained on them, detecting subtle deviations that traditional supervised models might miss.
Digital Twins with Simulation Capabilities. AI-enhanced digital twins now incorporate physics-based modeling with data-driven insights, enabling what-if scenario planning and predictive simulations that go beyond statistical forecasting.
Federated Learning. Organizations can now train models across distributed IoT networks without centralizing sensitive data, maintaining privacy while leveraging insights from multiple facilities or equipment fleets.
Explainable AI. AI-enabled solutions add context to raw sensor data, providing interpretable insights about predicted failures and helping maintenance teams understand not just when but why equipment might fail.
Prescriptive Maintenance. Beyond just predicting failures, modern AI systems recommend specific maintenance actions and optimize maintenance scheduling across entire asset portfolios.
Strategic Implications for Business Leaders
For executives navigating this shifting landscape, several strategic considerations emerge.
First, we have to reimagine data governance. Traditional data governance frameworks focused primarily on storage, security, and compliance. Today’s environments require governance models that address the ethical implications of automated decision-making and establish clear boundaries for AI autonomy.
Second, the scope of skills needed has evolved. The convergence of AI and IoT creates demand for hybrid talent—professionals who understand both domains and can bridge the gap between them. Organizations must either develop this talent internally or forge strategic partnerships to access it.
Finally, AIoT is disrupting value chains. As decisions move from human operators to automated systems, entire value chains are being reconfigured. Business leaders must evaluate how these changes impact their competitive positioning and customer relationships.
Looking Ahead: The Next Frontier
The integration of real-time AI and IoT is just beginning. As these technologies mature, we can expect to see increasingly sophisticated applications emerging across industries. The transformation isn’t just technical. It’s reshaping business models such as equipment-as-a-service offerings (EaaS), where AI-powered IoT enables performance-based contracts with guaranteed uptime rather than traditional maintenance arrangements.
The most forward-thinking organizations are already exploring concepts like collaborative intelligence networks, where multiple AI-powered IoT systems share insights and coordinate responses across organizational boundaries.
The companies that thrive in this new environment will be those that view real-time AI not merely as a technical enhancement but as a strategic imperative—one that enables them to reimagine their operations, customer experiences, and business models.
For business leaders, the message is clear: The future belongs to those who can harness the power of real-time intelligence to create systems that don’t just collect data but act on it autonomously, continuously, and intelligently.