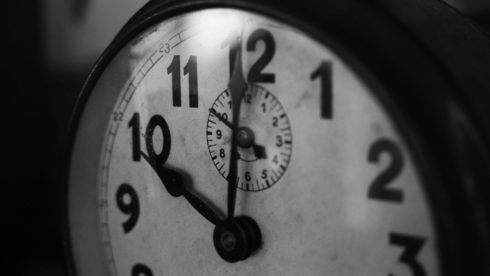
Predictive analytics technologies have become critical to compete in manufacturing (predicting machine failure), banking (predicting fraud), e-commerce (predicting buying behavior) as well as to address horizontal use cases such as cybersecurity breach prevention and sales forecasting. Using data to predict and prevent IT outages and issues is also a growing best practice—especially as advances in monitoring software have made it easier to deliver analytics in a timely manner.
The process of rapidly collecting IT systems data, including by integrating data from various monitoring tools in use, has become rudimentary. The magic is in the algorithms, which can deliver actionable intelligence. IT predictive analytics, once known as IT operations analytics (ITOA), is still nascent in many organizations, but it’s far more streamlined than it used to be, when one needed to export data sets to specialized analytics tools such as Tableau or Microsoft PowerBI. Data analysts and then data scientists were necessary to interact with these advanced tools and deliver valuable predictions – a process that could take weeks.
Today, IT professionals can use modern IT operations monitoring and management systems for collecting and integrating the data, normalizing it and then analyzing it in real-time. That’s making predictive analytics in IT far more user-friendly, affordable and useful for all organizations – not just the largest companies with the biggest budgets.
Advances in predictive analytics technology, aided by machine learning and AI, are creating more proactive workflows for IT operations teams. For instance:
- Storage usage. It is not a happy moment when IT finds out that the expensive storage array is down. It’s not enough to know after the fact that storage volumes were steadily climbing out of the normal pattern. By knowing ahead of time that a trend is heading out of the acceptable range, IT can research the cause and fix it before any damage is done. With cloud storage, risks include slow connectivity if network bandwidth is insufficient, or security breaches if the cloud provider’s security mechanisms are not sufficient for your use case. Predictive analytics can alert on these possibilities in time to take corrective action.
- Cloud-to-cloud performance comparison. An important aspect of predictive analytics is the ability to make correlations and show relationships between multiple data points. IT leaders are interested in learning about an application’s performance across the entire IT environment – which may include comparing historical performance across multiple clouds to predict weaknesses in one service over the other and mitigate them proactively. This use of predictive analysis could also be valuable in helping companies choose one cloud provider over another for particular applications and workloads.
- Container optimization. Making correlations is especially valuable when it comes to managing performance in a microservices environment. Collecting metrics for a containerized application isn’t useful by looking at each container per se, but it is useful when looking across the container environment (a.k.a. Kubernetes) to see if there are common metrics dangerously trending the wrong way across many or all containers.
A few tenets for predictive analytics in IT operations
Regardless of the tools that you choose to create a predictive analytics practice in your organization, there are several things you must get right first. Here are six recommendations to consider:
- Centralize the data into one or two systems at the most. This allows for data cleansing, normalization and a single (ideally) system of record for all other users and integrations.
- Invest in first-class data visualization. As business units now interact with IT management systems to see departmental usage and troubleshoot minor issues, tools should be user-friendly for people who are not data analysts. Dashboards and charts can tell the story simply and explain the correlations and conclusions that the software delivers.
- Make it actionable. There is no use in creating predictive data if it’s not automatically sent to the right individuals and/or shared with other systems to execute actions when needed. Think about it the Amazon way: we all know that people aren’t sitting behind monitors sending millions of individualized recommendations to customers every day. Your IT operations portfolio should behave the same way: automated, intelligent, helpful and always adapting to new information.
- Correlate data at the speed of IT change. Before there was such a thing as virtualization and cloud infrastructure, there weren’t many devices to monitor and things didn’t change much day-to-day. You could actually “set and forget” data center infrastructure components for weeks. Now, of course, changes are happening behind the scenes every minute without our knowledge. Yet your monitoring and change management tools need to know about it. By using standard deviation for change detection monitoring—four-hour increments are typical – you can ensure that your team always has an updated understanding of infrastructure status as well as the relationships between the various systems and components.
- Avoid the black box. Enrich your data lake continuously with event data and allow users to see the IT operations platform working in real time. With machine learning and artificial intelligence, there’s always a measure of doubt and a margin of error. This uncertainty can be counteracted by sending more diverse data sets so that the user can make her own conclusions. As well, when the machine makes an error in judgment, train it to avoid making the same mistake again.
- Update internal IT skills. You’ve heard it before. In the age of big data, IT organizations need data science expertise and skills. It’s important to acquire or develop these skills so that your team can combine machine intelligence with human insight and make better decisions. That is where AI-driven analytics holds the most promise.
We are just getting started on this new age of predictive analytics in IT operations. Before long, organizations will move from predicting down time and serious application issues to driving infrastructure optimization and cost savings. IT leaders will be able to answer with confidence, how much cloud infrastructure needs to be provisioned or deprovisioned over time. They will understand with better clarity common application scaling patterns, as well as overall capacity management needs. As predictive analytics takes hold, IT leaders will need to determine how to reorganize employees who previously spent hours scanning data on screens into more strategic roles.