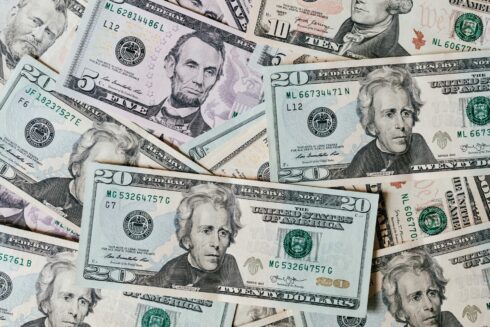
Enterprise AI is a broad topic, ranging from predictive analytics to generative AI, robotics, image recognition, natural language processing (NLP), chatbots and machine learning-based applications that perform complex tasks more efficiently and accurately than humans. Think AI on the manufacturing line to predict and prevent machine failures. Or AI in a nursing home to alert caregivers when a patient’s vitals show signs of a dangerous shift.
The flip side is that AI can be an expensive proposition—from creating the right infrastructure to developing and supporting AI applications, training learning models, deploying governance and security, conducting employee training, acquiring the needed IT skills to support AI and more.
In the 2024 Komprise State of Unstructured Data Management report, enterprise IT participants were asked how they would pay for AI and only 30% stated they will increase the budget for AI. One third (34%) will use their existing cloud services funds to pay for it. Merging AI into the cloud budget makes sense on one level, as the major cloud providers are all investing heavily in AI and ML technologies. Yet this may not be a panacea. High cloud costs and surprise bills have in many cases resulted in repatriation back on-premises after hefty cloud infrastructure investments during the pandemic.
Cost optimization is a central theme in IT in 2024: in fact, it was the top data storage priority in the Komprise survey. This is not a surprise, given that most survey participants are storing 5PB or more of unstructured data and say they’ll spend more on storage next year. By optimizing storage through data management, investing in energy-efficient data center technologies and being strategic with cloud spending versus “lift and shift,” organizations can find a budget to support revenue-generating AI initiatives.
The following considerations for deploying AI on a tight budget can ensure a smart path forward to delivering new value from petabytes of unstructured data:
- Determine the potential: With so many AI services being introduced, it would be easy to simply try a few and see what happens. But that usually isn’t the best way to adopt a new technology—plus it’s risky. A top-down approach involving senior leaders from business and IT along with stakeholders from legal, security, HR and compliance teams can help vet the opportunities and choose ones that have the lowest risk. Starting with just one AI project is a way to establish best practices that work for your organization including how to best leverage existing resources.
- Use cloud-based AI services: A logical step to get started on AI initiatives is to use AI services from AWS, Azure, Google, IBM and other cloud service providers (CSPs). These companies offer pre-trained AI models and APIs that can be fine-tuned and used for most internal projects such as natural language processing, image recognition, PII and other sensitive data detection and predictive analytics. The hyperscaler cloud providers offer GPU-based infrastructure with the horsepower needed to efficiently train and run AI models. Procuring, installing and supporting these high-end systems may not be affordable for the average IT department and may also require specialized skills. For enterprises with expensive AI initiatives and distinct security needs, however, it may end up being cheaper in the long run to house the technology in-house.
- Manage data over its lifecycle to free up budget: AI costs can be offset by efficient unstructured data management tactics which move data to lower cost storage (such as cloud object storage) as it ages and is rarely-accessed. Data tiering can eliminate the need for multiple backup and DR copies of all data, which makes up the largest portion of annual storage costs. Data lifecycle management also entails deleting data when it’s no longer required to retain along with finding and eliminating duplicate data. Analyzing data across storage silos regularly and the costs to store and protect it ensure that you are being as efficient as possible with storage—and thereby freeing up funds for AI.
- Green the data center: Green tech is nothing new, but progress remains slow. Yet the time is now and renewable technology prices are falling, according to the International Renewable Energy Agency. In 2022, new onshore wind project costs were 52% lower than the cheapest fossil fuel-fired solutions while solar power cost 29% less than the cheapest fossil fuel-fired solution. The 2022 U.S. Inflation Reduction Act is expected to further bring down costs of renewables from tax credits and other incentives. More states are rolling out energy-efficient mandates as well—further priming the pump. The good news is that when it’s time to upgrade servers, networks, storage and other hardware, the latest technologies are designed to be more efficient. Finally, by managing data independently of storage (per previous point) organizations can further cut energy usage by storing less active data–which is on average 80% of all data–on lower cost, lower-performing technologies which require less power.
- Experiment with AI-as-a-Service: There are many out-of-the-box AI solutions from both CSPs and software-as a service (SaaS) vendors that make setting up and maintaining AI much easier and may require minimal AI expertise. For general-purpose AI programs, an AIaaS can be a relatively low risk way to get started in AI. These solutions often eliminate the need to purchase specialized hardware since they are hosted by the vendor.
- AI-powered SaaS solutions: Increasingly, business SaaS vendors now incorporate AI features—across CRM, marketing applications, HR, finance and supply chain. So rather than developing and supporting a customized AI solution, an organization can get significant benefit simply from buying the latest versions of top products on the market. Again, this is a great way to learn more about AI and its potential before jumping in with larger investments.
- Adopt no-code or low-code AI platforms: These technologies claim to allow non-technical staff to build and deploy AI models without extensive coding knowledge. When looking at applications that have built-in AI or integrate with AI services, be sure to understand how easy it is to create and customize the AI capabilities without having coding or specific AI knowledge and skills.
Preparing for AI is a top business priority, according to the Komprise survey. Developing organizational best practices for AI might feel daunting, but there are simple ways to start experimenting with AI. Look for low-cost ways to leverage the cloud, easy low-risk projects to get started and avoid heavy customization initially. Data governance and automating unstructured data management can be crucial in reducing risk, especially in highly regulated industries.
You may also like…
Q&A: Is nuclear energy the key to meeting the demands of AI workloads?
Data scientists and developers need a better working relationship for AI