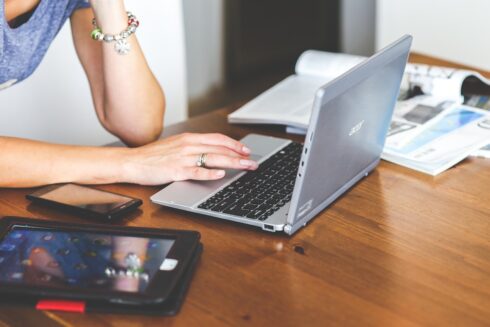
The artificial intelligence being used in operations already is advanced, with RPA (robotic process automation) and other rules-governed systems helping organizations become more efficient.
One area that is being looked at now is generative AI. According to Josh Mayfield, senior director of product marketing at Kentik, thinks about it like this: “Like a surveyor, you’re exploring with intent, to specifically take measurements for the viability of X, and being deliberate about what your plan of attack is going to be. Go and survey those various AI tools and then see how they fit with what you’re trying to get done.”
A second point he makes is to be patient when AI gets something wrong, or just not quite right. “Telling it what it got wrong really does improve it,” Mayfield said. “That’s the interesting thing about these tools, is they have this self-improvement that happens in the moment, far greater than running another model for machine learning or big data sets on more rules and workflows.”
RELATED:
A systemic approach to network observability
A guide to network observability tools
The problem with that approach is that when it gets something wrong, it’s a big lift to make sure it doesn’t make that mistake again. But with generative AI, it can get it right on the very next try.
Then, as you explore alternative solutions, it’s important to make sure how your data is used, because even your prompt questions can have a confidential nature to them. “Some vendors will say that don’t use your data to train on, but you’re still prompting it. If that ever leaked, it could indicate someone knows what you’re doing there.” Mayfield explained. It’s important, he added, to ensure that the back-and-forth of prompt and response is confidential, “because it’s the same as if you’re talking to a peer or colleague about something confidential inside your own organization.”
The last point Mayfield made is to be creative with AI, because there are things these tools can do with “just a little bit of nudging, and you may not even be prepared for what it will unleash and do.” AI is fed data and starts to learn about workflows, habits and more. First, it learns something in particular and then it starts to learn about adjacent results. Mayfield gave this example: “I asked it a question on Tuesday, go fetch me this cloud-to-cloud traffic for XYZ. It got some results. And I go and move along in my kinetic investigation. I click around a couple of things and it renders that chart. Okay. Now today I do the same thing. And it comes back with the same result. But it has a little extra, saying I thought you might also want to look at this chart. Because I’ve seen that through my investigations, that tends to be something else I’m going to incorporate.”
In this instance AI becomes a sort of digital intern that is learning as it goes. “So be creative about what you do with it, and just push the envelope of what you think might be learnable. If you think about it more like an intern, well, an intern can learn just about anything,” he said. “Think about what it might want to learn, and what you can teach it, and not strictly limit it to ‘inside the box.’ “