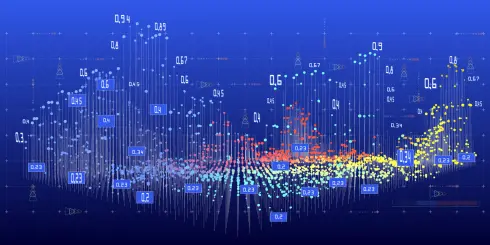
The rise of Artificial Intelligence (AI) is transforming industries across the globe, and the networking landscape is no exception. Network operations teams need to contend with new AI-centric workloads that use the network in novel ways. In this article, I draw insights from a recent discussion on this topic with Kamran Naqvi, Broadcom’s chief network architect for EMEA. We discussed the profound impact of AI on networks in 2025.
Networks for AI: Adapting to New Demands
AI workloads place unique demands on networks. Unlike traditional cloud computing, which involves numerous short-lived connections, AI applications generate a smaller number of “elephant flows” – sustained high-bandwidth communication between GPUs. These persistent connections necessitate a fundamental shift in network design, emphasizing low latency and high bandwidth to ensure efficient data transfer between processing units.
Furthermore, AI workloads are highly sensitive to packet loss. The use of technologies like Remote Direct Memory Access (RDMA) exacerbates this sensitivity, as a single packet drop can trigger significant retransmissions, impacting overall performance. This underscores the critical need for robust and reliable network infrastructure to support demanding AI applications.
Tail Latency: A Key Performance Bottleneck
The concept of “tail latency” is emerging as a crucial factor in AI performance. In iterative training processes, a single slow connection can significantly delay the entire process, impacting overall efficiency. This emphasizes the importance of network optimization to minimize latency and ensure all connections contribute effectively to the training process.
AI for Networks: Enhancing Observability and Automation
Understanding the operational characteristics of AI workloads and the impact on networks shifts our discussion to the application of AI within network operations. For AI to help with the operational aspect of monitoring and managing AI workloads, it needs to operate on valid, useful and clean data. High-quality data from your network observability solution has a critical role in enabling effective AI-driven solutions. “Garbage in, garbage out” aptly describes the limitations of AI models trained on incomplete or inaccurate data.
It’s important to have comprehensive network observability, encompassing granular flow data, detailed log messages, and a 360-degree view of the network. This data-driven approach enables AI algorithms to identify anomalies, predict potential issues, and automate network management tasks.
Self-Healing Networks: A Glimpse of the Future
The concept of “self-healing networks” has emerged as a key area of focus. By leveraging AI and machine learning, networks can proactively identify and resolve issues, such as network congestion or security breaches, before they significantly impact performance.
This involves utilizing advanced telemetry features like “mirror on drop,” which provides insights into the root causes of packet drops. By analyzing this data, AI algorithms can pinpoint the issue (e.g., missing VLAN configurations) and automatically implement corrective actions, such as reconfiguring network settings.
The Importance of Open Standards and Collaboration
For organizations to be successful with AI, open standards and collaboration within the industry are needed. For example, the Ultra Ethernet Consortium (UEC) has an important role in driving the evolution of Ethernet to support the demands of AI workloads. By fostering collaboration among industry players, UEC aims to ensure that the future of networking remains open and interoperable, avoiding the pitfalls of vendor lock-in.
Conclusion
The impact of AI on networks is profound and multifaceted. As AI workloads become increasingly prevalent, network infrastructure must evolve to meet the unique demands of these applications. By embracing AI-driven solutions, leveraging comprehensive network observability, and fostering collaboration within the industry, network operators can unlock the full potential of AI and build more intelligent, resilient, and efficient networks for the future.
I invite you to take a look at this eBook and learn 10 Ways to Future Proof Today’s Network Infrastructure for Tomorrow’s AI Workloads.